Introduction
In the context of globalization, language barriers remain a significant challenge for immigrants, international remote workers, and cross-border business professionals. In daily social interactions, business meetings, and online collaboration, even those fluent in foreign languages find it difficult to communicate as naturally as in their mother tongue. The rise of large language models (such as ChatGPT) has made real-time spoken translation across languages possible. How to “tame” a general-purpose conversational model and focus it on spoken translation tasks, combining it with dedicated hardware to create a reliable, low-latency AI simultaneous interpreting assistant, is a strategically important topic. This article explores the current state and future prospects of this field from five dimensions: model optimization, software-hardware integration advantages, key technical challenges, the outlook for human-like communication experience, and the optimal future technology roadmap. It aims to provide strategic thinking and technical insights for entrepreneurs, AI product designers, and visionary investors.
1. Specializing Large Models: Translation Mode Optimization and Latency Reduction
General chat models like ChatGPT have multilingual translation abilities, but to become a professional spoken translation engine, they need to be optimized and trimmed for translation tasks.
(1) Fine-tuning and specialized training: By fine-tuning models on large-scale parallel corpora, translation accuracy and terminology consistency can be greatly improved.
(2) Model compression and pruning: To reduce translation latency, techniques such as model distillation can be used to train lightweight models that approximate the translation capabilities of large models, thus reducing inference overhead. Newer, smaller models emphasize low latency and faster response times, suitable for tasks with high speed requirements. By customizing models for specific uses, unnecessary computational overhead can be avoided, further reducing average latency.
(3) Explicit role instructions and output constraints: At the application level, strict system prompts can be imposed to restrict ChatGPT to the role of translator, preventing it from engaging in small talk or generating content beyond translation. This instruction-following ability has been greatly improved in the latest versions, enabling the model to reliably stick to preset tasks. By setting a fixed prompt at the start of each session (e.g., “translate only, no explanations”) and post-processing the output, the model can be kept focused on faithful translation, avoiding subjective comments or topic drift.
(4) Streaming translation and parallel processing: For a “simultaneous interpreting” experience, the model can receive speech-to-text input and output translations incrementally—streaming translation. This requires special decoding strategies to ensure that the segmented output is natural and coherent. Combining streaming ASR (automatic speech recognition) and segmented translation output can reduce the time waiting for an entire sentence, achieving small chunks of translation output every few seconds for better real-time performance.
In summary, with fine-tuning for reliability, slimming and architectural changes for lower latency, plus strict task constraints and streaming decoding strategies, large models like ChatGPT can achieve higher practicality and efficiency in translation applications.
2. Ten Advantages of the APP+Hardware Solution
Integrating an AI translation system with a suite of hardware—clip-on microphones, mobile phone speakers, earphones, and screens—brings many practical advantages, enabling users to communicate across languages in real time in various scenarios. Here are ten benefits of the APP+hardware approach:
- Private communication: Using earphones to output translated speech ensures that only the conversation parties can hear, without disturbing others.
- Hands-free operation: With clip-on microphones and wireless earphones, users can converse without holding devices, freeing their hands for note-taking or giving presentations and making communication more natural.
- Easy deployment: Most people already own smartphones; adding a small microphone and earphones creates a translation system at low cost. No complicated installation is needed—just install the app and pair devices via Bluetooth to enable real-time translation.
- Multi-scenario coverage: The APP+hardware combination supports multiple modes, suitable for nearly all communication scenarios: one-on-one conversations with earbuds, group meetings with phone speakers, subtitle display on screens in noisy environments, and on-the-go translation with earphones.
- Real-time translation: With cloud or on-device AI engines, the system can complete the speech recognition-translation-synthesis cycle within a couple of seconds, enabling nearly synchronous conversation.
- High accuracy: Modern AI translation, trained on large datasets, offers high accuracy in common contexts and minimizes misunderstandings. On-screen text allows both parties to verify content, further improving comprehension.
- Noise reduction: Clip-on microphones close to the speaker and built-in noise reduction algorithms significantly reduce background noise, ensuring clear voice input.
- Natural interaction: Hidden earphones and compact microphones allow participants to maintain eye contact and normal body language, making communication closer to face-to-face conversation.
- Multilingual support: The software can support dozens of languages and accents, making the system truly global.
- Cost-effectiveness: Compared to hiring human interpreters or buying expensive conference systems, the phone+accessory solution is low cost and scalable, with ongoing software updates improving performance.
In summary, the combination of mobile apps and wearable hardware brings portability, real-time performance, privacy, and ease of use to AI translation, turning the sci-fi dream of a “portable translator” into reality and providing a practical path to breaking down language barriers.
3. Ten Technical Challenges for Simultaneous Interpreting Applications
Despite promising prospects, achieving reliable and smooth real-time spoken translation requires overcoming many technical challenges. The following summarizes the ten main difficulties currently encountered in practice:
- Speech recognition errors: Errors in the speech input phase directly cause inaccurate translations. Accents, speaking speed, and word choice differences can cause ASR models to make mistakes. In noisy environments or with unclear pronunciation, the system may misinterpret the original speech.
- Translation latency: Latency disrupts real-time communication. If the system waits for a full sentence before translating, awkward pauses or interruptions may occur. While delays under two seconds are acceptable, longer delays break the conversation flow. Reducing end-to-end latency remains a technical challenge.
- Unnatural segmentation and connection: Current systems often translate by sentence, possibly splitting speech at inappropriate points and producing choppy or incoherent translations. If a speaker keeps talking in long sentences, the system may have to interrupt or wait a long time to output a translation, degrading the listening experience. Sentence structure differences between languages make real-time segmentation even more challenging.
- Background noise interference: In noisy environments, even humans struggle to hear, let alone machines. Although advanced devices have noise-cancelling microphones, recognition accuracy drops sharply in crowds or strong wind. Background noise affects not only ASR but may also trigger wake words or confuse the system.
- Cross-cultural misunderstandings: Machine translation struggles with idioms, slang, humor, and other culturally embedded content. Many expressions do not translate literally and can cause confusion or embarrassment. AI often fails to grasp the cultural meaning behind statements.
- Accents and dialects: Speakers from different regions have distinctive pronunciations, and the same language may have diverse dialects. Training data often favors standard accents, with insufficient coverage for minority dialects, leading to higher error rates when encountering them.
- Multilingual environment management: In some settings, more than two languages may be used, or conversations may mix languages. AI systems must detect the current language and switch translation targets, which adds complexity. Multiple speakers talking in different languages simultaneously makes correct separation and translation even harder. Determining which listener should receive which translation is also a challenge.
- Battery and computing power limitations: High-quality speech translation requires continuous heavy computation, which quickly drains batteries on mobile devices. Dedicated translation earbuds may need recharging after a few hours. Smartphones running translation apps may overheat or throttle performance during long use. If computing power is insufficient, the system may have to use local models with lower accuracy or simplified algorithms, affecting translation quality. Achieving long, stable operation under power constraints remains difficult.
- Network reliance and data usage: The best ASR and translation models typically run in the cloud, requiring network connectivity. Poor or unavailable networks degrade system performance. Some scenarios require privacy, making cloud-based translation unsuitable. Balancing online and offline modes is challenging—cloud models offer higher accuracy but risk latency and privacy issues; offline models are more private but less capable. Leveraging the power of large models while ensuring privacy is a challenge.
- Context memory and consistency: Dialogue is continuous, but machines often lack context memory, translating each sentence independently, causing pronoun and terminology inconsistency. For example, pronouns in one sentence may be mistranslated in the next, or key terms may be translated differently throughout a conversation. Giving models cross-sentence context understanding requires more advanced algorithms and memory, which is still being explored.
These challenges reflect the complexity of simultaneous machine translation, which brings together speech recognition, natural language understanding and generation, and multimodal processing—each with its own bottlenecks. Some problems can be gradually alleviated with better data and algorithms, such as noise adaptation and accent coverage, but others are deep-seated linguistic and cultural issues that are difficult to solve in the short term. On the path to perfect solutions, we need to face and tackle these bottlenecks one by one.
4. Native-Like Communication Experience: Hope and Cognitive Barriers
Is it possible to use AI to achieve cross-language communication as smooth and natural as a native conversation? This is a beautiful vision for many. Current progress brings hope: top systems are rapidly approaching human interpreter levels in speed and accuracy. Some new technologies are narrowing the gap between machine and human translation experiences. For example, some end-to-end speech-to-speech translation systems can preserve the speaker’s vocal characteristics, making it sound as if the original person is speaking in another language. Combining with technologies like AR subtitles, some early experiments already show near sci-fi effects: wearing smart glasses, users can see real-time translation subtitles in front of their eyes, greatly reducing communication barriers.
However, to truly attain a “native-level” communication experience, AI faces some insurmountable cognitive obstacles. The most important are the transmission of tone and emotion—language is not just information exchange, but also carries emotional color and attitude. Human interpreters strive to restore emotion in translation, but machines lack emotional intelligence and often produce flat, tone-deaf translations. For passionate speeches, AI may render the literal meaning, but cannot reproduce the same emotional impact. Another shortcoming is understanding cultural subtext. Much of language’s meaning is implicit and depends on common knowledge and cultural context. Human translators consider context and audience background to adjust wording and avoid literal translation that could cause misunderstanding or offense. AI, lacking social awareness, often ignores such nuances, leading to awkward results. Humor, sarcasm, and wordplay are even harder for machines—without life experience, AI translates jokes literally, often causing confusion rather than amusement.
There are also subtle aspects of human communication—artful pauses, degrees of politeness, etiquette—that are deeply rooted in culture and require empathy and social awareness. While AI can be programmed with some rules, it is nearly impossible to cover all the unwritten rules for every scenario.
In summary, in the foreseeable future, AI may match humans in conveying information, but there remains an unbridgeable gap in conveying subtext and emotion. Truly “barrier-free, native-like” communication may require general AI to reach human cognitive levels. Thus, a realistic expectation is that AI translation will greatly reduce language barriers and make cross-language communication extraordinarily convenient, but achieving the same experience as native communication remains out of reach. Some subtle but important human elements—shared laughter, empathetic comfort, understanding implied meaning—still require human wisdom. For the foreseeable future, a combination of “AI + human” may be the optimal path: AI provides fast translation, while humans polish and adjust for emotional and cultural fit, retaining the irreplaceable value of human interpreters in complex situations.
5. The Future of Wearable AI Translation Assistants: Technical Prospects
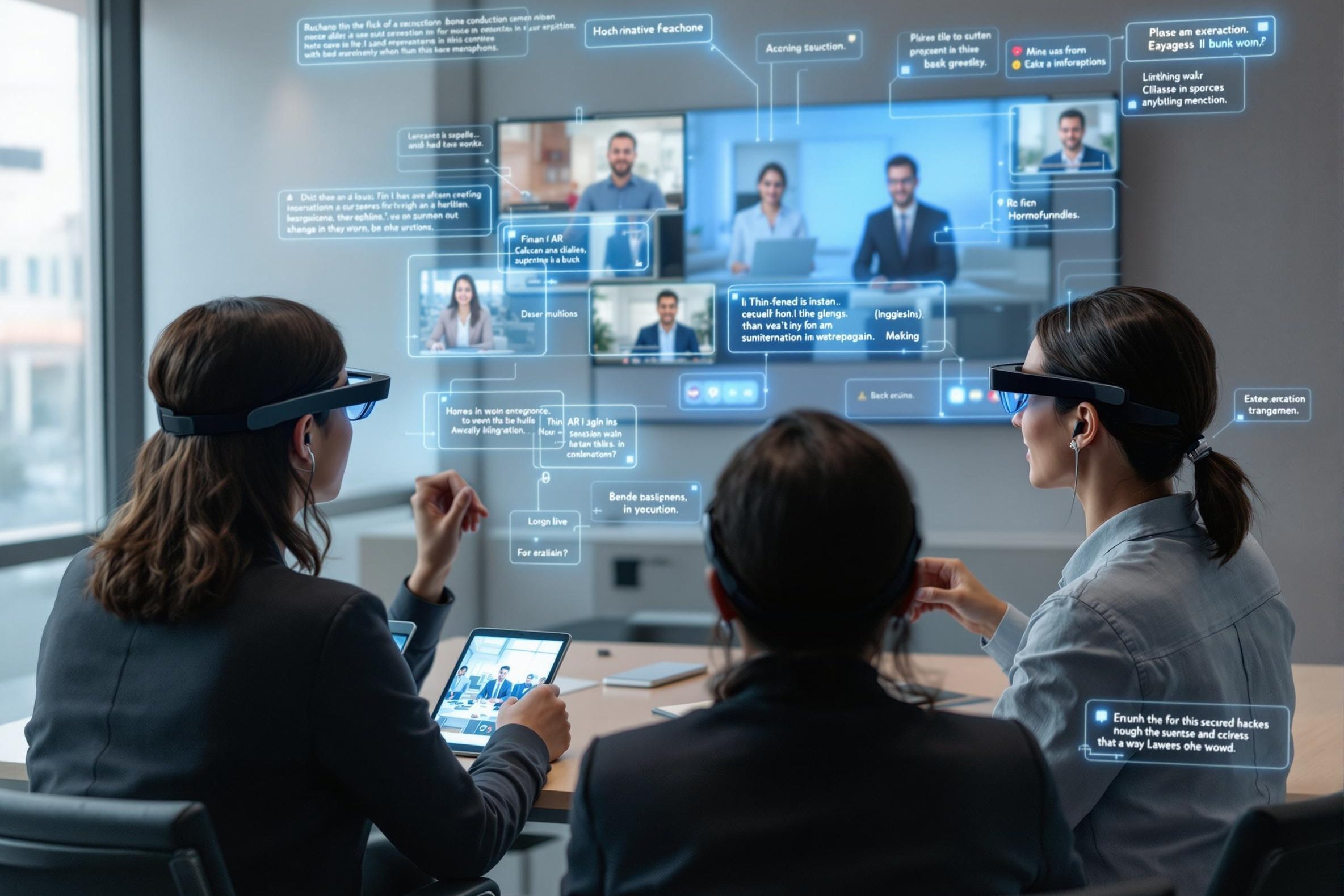
Looking to the future, achieving a truly wearable AI translation assistant will likely require the integration of multiple cutting-edge technologies to create a seamless and coordinated solution:
- On-device inference and dedicated chips: Future translation assistants will rely more on local processing power, enabling offline real-time translation and avoiding network dependence. With the development of dedicated NPUs/DSPs, phones and earbuds will be able to run large models or distilled versions locally, completing the entire process on-device. This not only increases speed and privacy but also enables users in remote areas to use translation without internet access. Translation algorithms will be deeply integrated into mobile SoCs, operating as efficiently as today’s fingerprint chips.
- Low-power microphone arrays: Future devices may use array microphones for improved voice capture, while maintaining ultra-low power consumption and tiny form factors. Beamforming technology can focus on the speaker’s voice and filter out background noise. The key is to integrate these into compact wearable devices such as earbuds or necklaces while ensuring low power consumption. For example, some translation earbuds already use dual-microphone noise cancellation; future devices may have 4-6 MEMS microphones in a collar or glasses, achieving 360-degree voice capture and noise filtering.
- Bone conduction headphones: These allow users to hear AI translations while still perceiving ambient sounds, providing comfort and safety for long-term use. This means users can hear both the original speech through their ears and the translation via bone conduction, making communication more natural.
- Real-time subtitles and AR glasses: Future translation assistants are likely to include visual output, such as AR glasses projecting real-time subtitles. Wearers can see translated text in front of their eyes during conversation, just like watching movie subtitles. This benefits both hearing and hearing-impaired users. As AR glasses become lighter and more affordable, business communication across languages may only require wearing smart glasses and a tiny earpiece for seamless, sci-fi-like communication.
- Personalized local models: With privacy computing and local deployment becoming trends, every user may have a private translation model on their device, constantly fine-tuned to their language style and terminology. For example, a doctor’s assistant model may be specially trained in medical terms, while a diplomat’s model learns multilingual etiquette. These models can remember frequently used names and places, continuously optimizing translation using personal glossaries. With federated learning, user devices can share model improvements without exposing data.
- Direct speech-to-speech translation: The optimal future solution may use end-to-end S2ST models, mapping input speech directly to another language’s speech while preserving speaker characteristics, further reducing latency and compounding errors.
- Multimodal and environmental awareness: Truly intelligent assistants will integrate audio with visual and environmental cues (such as facial expressions, gestures, or projected slides) for more precise translation. For example, recognizing who is speaking and directing translated output to the right listener’s device. Environmental awareness will help adjust translation style for formal or informal settings.
- High security and reliability: In business and government scenarios, translation assistants must be secure and reliable. Future systems will feature local encryption to prevent eavesdropping, rigorous validation to avoid mistranslations, and automatic fallback modes for unstable connections or limited resources.
Based on these elements, we can envision the optimal future solution: a businessperson wearing stylish, lightweight AR glasses, with bone conduction headphones and a miniature microphone array. This setup, powered by a local large model, listens to others’ speech, displays subtitles via the glasses, provides synchronized audio translation via headphones, and translates the user’s speech for others’ devices. The entire process works offline, even on a plane. The AI can infer discussion topics and file references for accurate, context-appropriate translation. Both parties feel almost no language barrier—the experience is close to monolingual communication, with AI working behind the scenes. This “perfect simultaneous interpreter” will combine powerful edge AI chips, advanced speech algorithms, and human-centered device design. While realizing this vision requires overcoming many engineering challenges, the value is immense: breaking language barriers and enabling seamless global communication.
Conclusion
Starting from large models like ChatGPT and customizing them for specialized tasks, combined with refined hardware and system design, we are getting closer to the vision of “talking to anyone with wearable equipment.” Opportunities and challenges coexist: on one hand, technological advances are rapidly closing the gap in translation quality and experience; on the other, emotional and cultural barriers remind us of the unique value of human wisdom. For entrepreneurs and product designers, focusing on this field requires both cutting-edge AI innovation and deep insight into the essence of human communication. In the foreseeable future, AI spoken translation assistants will continue to evolve towards faster, deeper understanding and more natural experiences, becoming another vital tool for connecting the world. As key technologies mature, wearable AI interpreters will no longer be confined to science fiction—they will enter our lives, empowering every cross-language conversation and ensuring that every voice in the “global village” is heard and understood.
Leave a Reply